You are here
- Home
- Makram HATOUM : "Digital watermarking for PDF documents and images: security, robustness and AI-based attack"
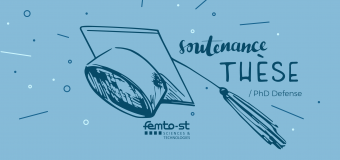
Makram HATOUM : "Digital watermarking for PDF documents and images: security, robustness and AI-based attack"
Wednesday 23 september 2020 - 9.00 A.MPhD works of Makram Hatoum :"Digital watermarking for PDF documents and images: security, robustness and AI-based attack"
Abstract : Technological development has its pros and cons. Nowadays, we can easily share, download, and upload digital content using the Internet. Also, malicious users can illegally change, duplicate, and distribute any kind of information, such as images and documents. Therefore, we should protect such contents and arrest the perpetrator. The goal of this thesis is to protect PDF documents and images using the Spread Transform Dither Modulation (STDM), as a digital watermarking technique, while taking into consideration the main requirements of transparency, robustness, and security.
STDM watermarking scheme achieved a good level of transparency and robustness against noise attacks. The key to this scheme is the projection vector that aims to spreads the embedded message over a set of cover elements. However, such a key vector can be estimated by unauthorized users using the Blind Source Separation (BSS) techniques. In our first contribution, we present our proposed CAR-STDM (Component Analysis Resistant-STDM) watermarking scheme, which guarantees security while preserving the transparency and robustness against noise attacks.
STDM is also affected by the Fixed Gain Attack (FGA). In the second contribution, we present our proposed N-STDM watermarking scheme that resists the FGA attack and enhances the robustness against the Additive White Gaussian Noise (AWGN) attack, JPEG compression attack, and variety of filtering and geometric attacks. Experimentations have been conducted distinctly on PDF documents and images in the spatial domain and frequency domain.
Recently, Deep Learning and Neural Networks achieved noticeable development and improvement, especially in image processing, segmentation, and classification. Diverse models such as Convolutional Neural Network (CNN) are exploited for modeling image priors for denoising. CNN has a suitable denoising performance, and it could be harmful to watermarked images. In the third contribution, we present the effect of a Fully Convolutional Neural Network (FCNN), as a denoising attack, on watermarked images. STDM and Spread Spectrum (SS) are used as watermarking schemes to embed the watermarks in the images using several scenarios. This evaluation shows that such type of denoising attack preserves the image quality while breaking the robustness of all evaluated watermarked schemes.
Jury composition :
Jean-François COUCHOT, MCF, Université Bourgogne - Franche-Comté, PhD Director
Rony DARAZI, Professeur, Université Antonine, PhD CoDirector
Benoît MACQ, Professeur, Institute of Information and Communication Technologies, Electronics and Applied Mathematics, Reporter
Pierre SPITERI, Professeur des Universités, Institut National Polytechnique, Reporter
Sylvain CONTASSOT-VIVIER, Professeur des Universités, Institut National Polytechnique, Reporter
Localization : FEMTO-ST, département DISC IUT Belfort Montbéliard 19 Avenue du Maréchal Juin 90016 BELFORT - salle Amphithéâtre 4, Génie Civil