You are here
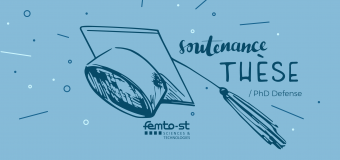
Wissam ALJURDI : "Towards New Generation Recommendation Systems thanks to Data Quality Generic"
Tuesday 26th March - 10.00 A.M.Abstract : Recommender systems are essential for filtering online information and delivering personalized content, thereby reducing the effort users need to find relevant information. They can be content-based, collaborative, or hybrid, each with a unique recommendation approach. These systems are crucial in various fields, including e-commerce, where they help customers find pertinent products, enhancing user experience and increasing sales. A significant aspect of these systems is the concept of unexpectedness, which involves discovering new and surprising items. This feature, while improving user engagement and experience, is complex and subjective, requiring a deep understanding of serendipitous recommendations for its measurement and optimization. Natural noise, an unpredictable data variation, can influence serendipity in recommender systems. It can introduce diversity and unexpectedness in recommendations, leading to pleasant surprises. However, it can also reduce recommendation relevance, causing user frustration. Therefore, it is crucial to design systems that balance natural noise and serendipity. Inconsistent user information due to natural noise can negatively impact recommender systems, leading to lower-quality recommendations. Current evaluation methods often overlook critical user-oriented factors, making noise detection a challenge. To provide powerful recommendations, it’s important to consider diverse user profiles, eliminate noise in datasets, and effectively present users with relevant content from vast data catalogs. This thesis emphasizes the role of serendipity in enhancing
recommender systems and preventing filter bubbles. It proposes serendipity-aware techniques to manage noise, identifies algorithm flaws, suggests a user-centric evaluation method, and proposes a community-based architecture for improved performance. It highlights the need for a system that balances serendipity and considers natural noise and other performance factors. The objectives, experiments, and tests aim to refine recommender systems and offer a versatile assessment approach.
Jury Composition :
M. Abdallah MAKHOUL Professeur des universités Université Bourgogne Franche-Comté, PhD Director
Mme Lynda MOKDAD Professeur des universités Université Paris-Est Val de Marne, Rapporteur
M. Mohamed-Hedi KARRAY Professeur des universités University of Technology of Tarbes (UTTOP), Rapporteur
M. Jacques DEMERJIAN Professeur des universités Université Libanaise, Co-directeur de thèse
Mme Nadia KABACHI Maître de conférences Université Lyon1, Examinatrice
M. Julien BOURGEOIS Professeur des universités Université Bourgogne Franche-Comté, Examinateur
M. Jacques BOU ABDO Assistant professor University of Cincinnati, Examinateur