You are here
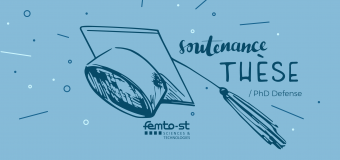
Zhiguang HUA : "Lifespan Prediction of Proton Exchange Membrane Fuel Cell System"
Wednesday 8 December 2021 - 2.00 P.MAbstract : The proton exchange membrane fuel cell is considered a promising power conversion device in different areas, nevertheless, the limited lifetime is one of the key barriers to their large-scale industrial deployment. The data-driven prognostic method aims to estimate the remaining useful life without the need for complete knowledge about the system’s physical phenomena. As an improved structure of the recurrent neural network, the echo state network has demonstrated better performances, especially in reducing the computational complexity and accelerating the convergence rate. To overcome the weakness of static health indicators commonly used (e.g., voltage and power), a convenient and practical health indicator named relative power-loss rate is proposed in this thesis. According to the polarization curve at the beginning of life, the initial power under different mission profiles can be identified. Then the actual power is obtained by monitoring the current and voltage continuously. Finally, the relative power-loss rate is calculated based on the initial power and actual power. A multi-input and multi-output echo state network structure is developed and tested for the steady-state, quasi-dynamic, and full dynamic operating conditions of the fuel cell. The echo state network with double inputs could imitate the degradation properties more sufficiently and improve the prediction precision under the dynamic operating conditions. Based on the relative power-loss rate indicator, a combined method, namely discrete wavelet transform and ensemble echo state network is proposed to deal with the multi-timescale features and improve the long-term prediction accuracy. A data-driven approach of discrete wavelet transform-echo state network-genetic algorithm is proposed to further improve the prediction performance. The performance of the proposed approaches is evaluated by different experimental datasets under steady-state, quasi-dynamic, and full dynamic operating conditions separately.
Jury Composition :
Fei GAO, Professeur des universités, Univ. Bourgogne Franche-Comté, UTBM,PhD Director
Loïc BOULON, Full professor, Université du Québec à Trois-Rivières, Reporter
Belkacem OULD BOUAMAMA , Professeur des universités, Université de Lille 1, Reporter
Christophe TURPIN, Directeur de recherche, Laplace laboratory , Reviewer
Nada ZAMEL, Docteure, Fraunhofer Institute for Solar Energy Systems ISE, Reviewer
Huizi WANG, Senior Lecturer, Imperial College London, Reviewer
Zhixue ZHENG, Maître de conférences, Université de Lorraine & CentraleSupélec, PhD Co-supervisor
Marie-Cécile PERA, Professeur des universités, Univ. Bourgogne Franche-Comté, UTBM, PhD Co-supervisor
Localization : UTBM, 13 Rue Thierry Mieg, 90010 Belfort Cedex, France - Room E107