You are here
- Home
- Zhihao CHEN : "Deep learning for automatic detection and quantification of the disease areas of the myocardium from DE-MRI after myocardial infarction"
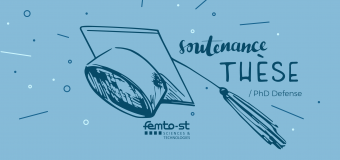
Zhihao CHEN : "Deep learning for automatic detection and quantification of the disease areas of the myocardium from DE-MRI after myocardial infarction"
Tuesday 19 October 2021 - 3.00 P.MAbstract : Myocardial Infarction (MI) has become one of the most common cardiovascular diseases. The MI occurs when the blood flow decreases or stops to a part of the heart which can cause damage to the myocardium due to ischaemia. The MI can lead to persistent microvascular obstruction (PMO) even after the reperfusion therapy of an occluded coronary artery, which affects a significant proportion of patients. To assess the MI and the extended PMO, Delayed Enhancement MRI (DE-MRI) has become the gold standard. However, to precisely quantify the pathology, physicians have to first manually draw the delineations of different myocardial tissues on the short-axis MRI slices then the volume of normal and pathological tissues will be calculated to evaluate the severity of the attack. The manual annotation procedures are highly time-consuming and subjective, which reinforces the potential interest of an automatic evaluation approach for the MI assessment. Current automatic delineation methods on DE-MRI are mostly probabilistic approaches such as the Mixture Models while more and more deep learning applications achieve state-of-the-art performances compared to the probabilistic approaches. Therefore, in this thesis, the different aspects of deep learning-based approaches have been investigated for the automatic MI evaluation from DE-MRI.
To develop the deep learning-based MI assessment pipeline, three major aspects consisting in the pre-processing, the deep learning models and the post-processing have been investigated or proposed. The pre-processing aims at preparing more consistent and clearer inputs for deep learnings models using image normalizations, cropping etc. To design well-adaptive deep learning models, many parts (building blocks, loss functions, segmentation models, etc.) were proposed or compared. Moreover, the post-processing methods including morphological treatments and prior information-based filters were applied to the coarse segmentations to eliminates false positive contours. Apart from the automatic assessment by segmentation, merging the MRI and textual patient features, a machine learning-based approach was proposed to obtain quantitative estimates of MI using only the clinical and pathological features for a quick cardiological assessment in cardiac emergency department.
Jury Composition :
Raphaël COUTURIER, Professeur, Université Bourgogne Franche-Comté, PHD Director
Nicolas PASSAT, Professeur, Université de Reims Champagne-Ardenne, CReSTIC, Reporter
Bruno KASTLER , Professeur, Service d'Imagerie adultes, Hôpital Necker-Enfants Malades, Reviewer
Pierre-Marc JODOIn, Professeur, Université de Sherbrooke, Reporter
Alain LALANDE, Maître de conférences des universités - clinician, Université Bourgogne Franche-Comté, PhD co-director
Michel SALOMON, Maître de conférences, Université Bourgogne Franche-Comté, PhD CoDirector
Localization : IUT Belfort-Montbéliard Building E - IT Department - 19 Avenue du Maréchal Juin 90016 Belfort (Room E8)